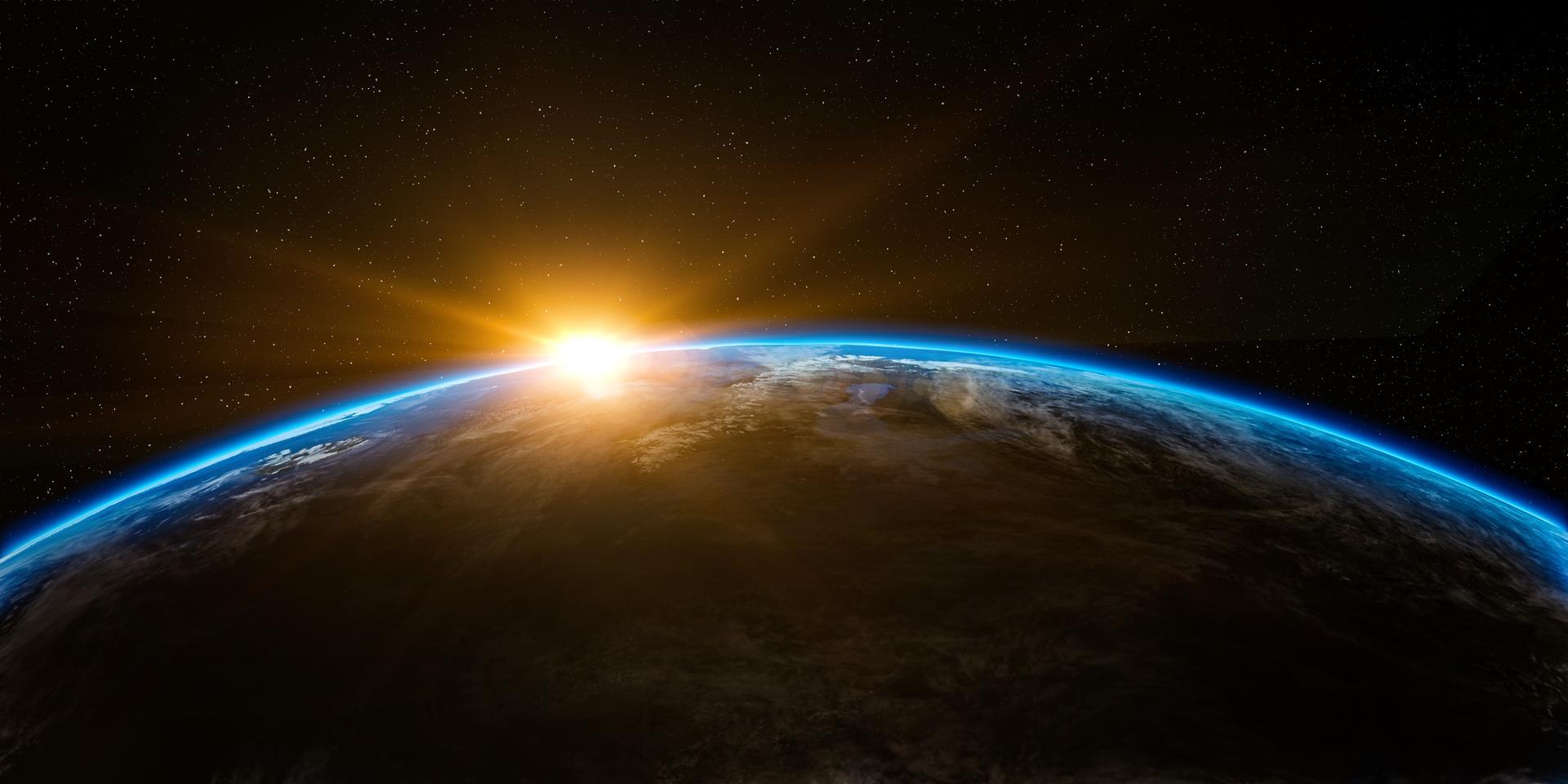
Unlocking the Power of Satellite Imagery with SeeFar: A Comprehensive Multi-Resolution Dataset
James Lowman and Mojtaba Valipour
June 10th, 2024
Dataset Link:
Paper Link:
In the rapidly evolving field of geospatial analysis, access to high-quality satellite imagery is paramount. The SeeFar dataset represents a significant leap forward by providing a multi-resolution, satellite-agnostic dataset designed to train geospatial foundation models. This blog post delves into the creation, significance, and potential applications of the SeeFar dataset, highlighting its contributions to the geospatial community.
Introduction to SeeFar
Satellite imagery has transformed our understanding of the Earth, offering invaluable data for environmental monitoring, urban planning, disaster response, and agricultural management. Advances in satellite technology have dramatically increased the accessibility and quality of this imagery. However, challenges such as high costs and limited historical availability of commercial satellite data persist, impeding the training of comprehensive geospatial models.
The SeeFar dataset addresses these challenges by curating a collection of multi-resolution satellite images from both public and commercial sources. This dataset is designed to be satellite-agnostic, enabling the integration of historical low-resolution data with modern high-resolution imagery, thus providing greater flexibility and accuracy during model inference.
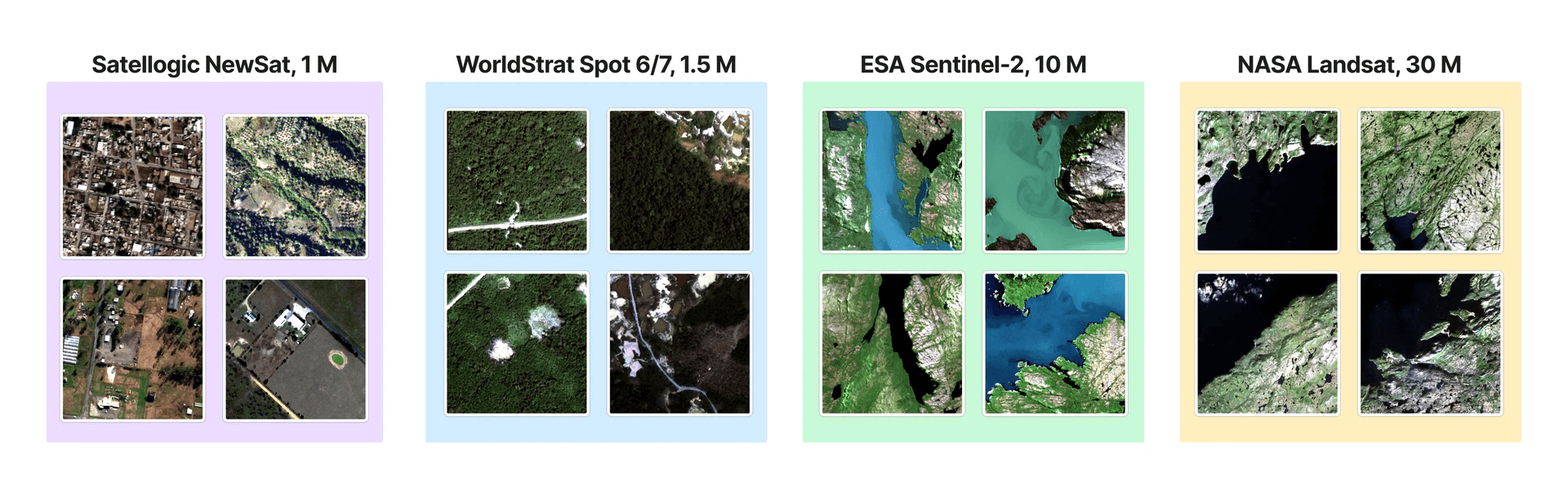
The Problem with Current Satellite Datasets
Existing satellite datasets face several limitations that hinder their effective integration and analysis:
- Multi-Format Data: The diversity in data formats makes it challenging to combine and analyze images from different sources.
- Inconsistent Metadata: Variability in metadata across datasets complicates tasks such as temporal analysis and multi-sensor fusion.
- Temporal Scale Differences: Differences in temporal resolution limit consistent time-series analysis.
- Non-Uniform Spectral Bands: Variations in spectral bands make data standardization difficult.
- Variable Resolutions: Discrepancies in spatial resolution affect the accuracy and reliability of multi-resolution analysis.
SeeFar aims to overcome these obstacles by standardizing data from diverse satellite sources, thereby enhancing its applicability and facilitating more robust and scalable research and applications.
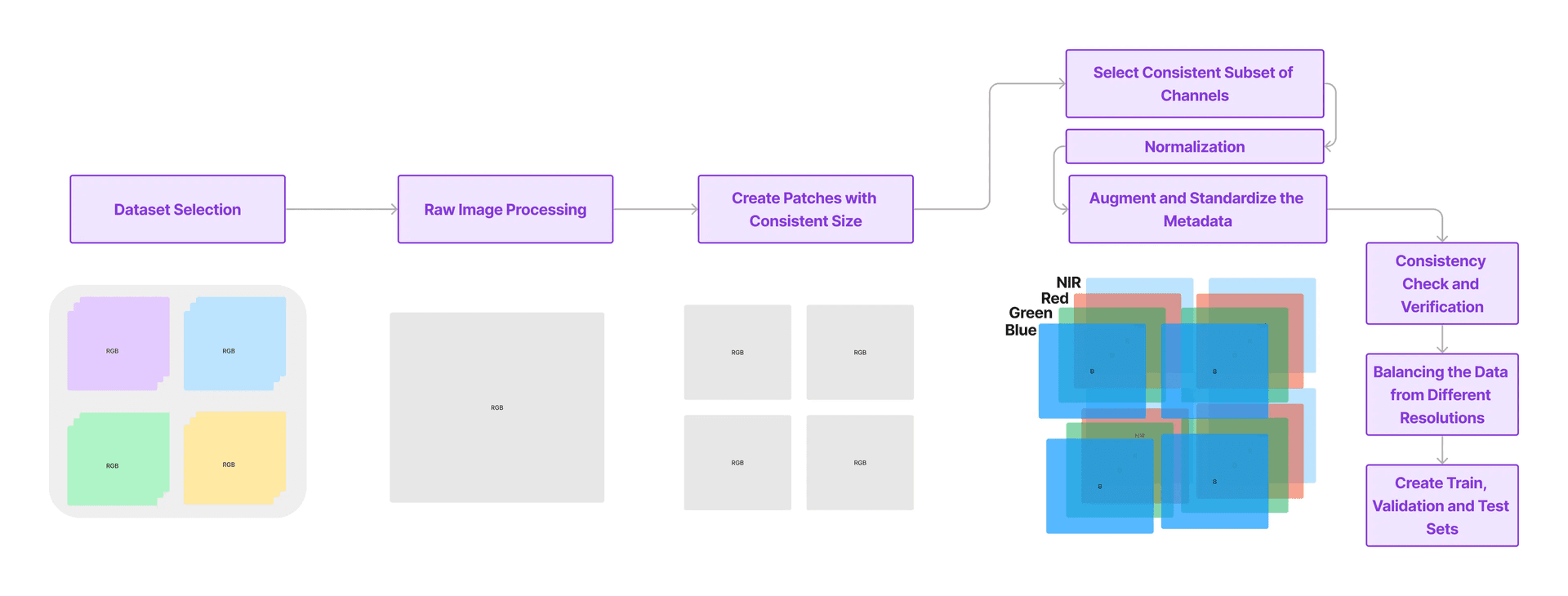
Key Contributions of SeeFar
The SeeFar dataset offers several critical contributions to the field of geospatial analysis:
- Facilitating Foundation Model Training: By integrating images from multiple resolutions and harmonizing multispectral bands, SeeFar supports the development of satellite-agnostic foundation models. This approach enhances the adaptability of models to various satellite imagery, both public and commercial.
- Standardization: A robust framework was developed to standardize data from various satellite sources, ensuring consistency and enhancing interoperability across different imagery.
- Benchmarking: SeeFar includes well-curated training, validation, and test sets, enabling the fine-tuning and evaluation of geospatial models. These benchmarks support the development of models capable of handling diverse satellite data.
- Multi-Resolution and Multi-Satellite Usability: The dataset consists of images at four different resolutions (30 meters, 10 meters, 1.5 meters, and 1 meter) from satellites such as Landsat 8 and 9, Sentinel 2, NewSat IV, and Spot 6 and 7. This multi-resolution approach supports a wide range of geospatial analysis tasks.
- Metadata Augmentation: Comprehensive metadata is provided, including Ground Sample Distance (GSD), spectral wavelength bounds, and georeferencing information. This metadata ensures data transparency and reliability, crucial for precise scientific analysis.
Methodology Behind SeeFar
The creation of the SeeFar dataset involved several meticulous steps to ensure consistency and quality:
- Dataset Selection: A subset of public and commercial datasets was selected based on licensing, content richness, alignment possibilities, and unique resolutions.
- Raw Image Processing: Each data source was pre-processed to ensure consistent information across different formats.
- Patch Creation: Consistent patch sizes (384x384 pixels) were created to focus on semantic differences rather than aspect ratios or other biases.
- Channel Selection: Blue, Green, Red, and Near-Infrared (NIR) spectral bands were selected for consistency across datasets.
- Normalization: Histogram matching was used to ensure consistent color, brightness, and contrast across different samples.
- Metadata Augmentation: Metadata was augmented to include GSD, spectral bands, and georeferencing information.
- Quality Control: Automated mechanisms were used to check for consistency and quality, including cloud and no-data pixel identification.
Conclusion and Future Directions
The SeeFar dataset represents a significant advancement in the field of geospatial analysis, offering a comprehensive and standardized dataset that bridges the gap between different satellite sources. By providing high-quality, multi-resolution satellite data, SeeFar enables more informed decision-making and supports a broad spectrum of geospatial research and applications.
As SeeFar continues to evolve, future updates and expansions will aim to incorporate a broader range of resolutions and further improve data quality and consistency. This ongoing development ensures that SeeFar will remain a valuable resource for researchers, policymakers, and industry professionals, fostering innovation and advancing the field of satellite imagery analysis.
In summary, SeeFar provides the geospatial community with a powerful tool for unlocking the full potential of satellite imagery, paving the way for more accurate, flexible, and scalable geospatial models and analyses.
Notes:
- Presented by Coastal Carbon AI Team.
- AI assistants has been utilized for enhancing the quality of writing.
recent articles
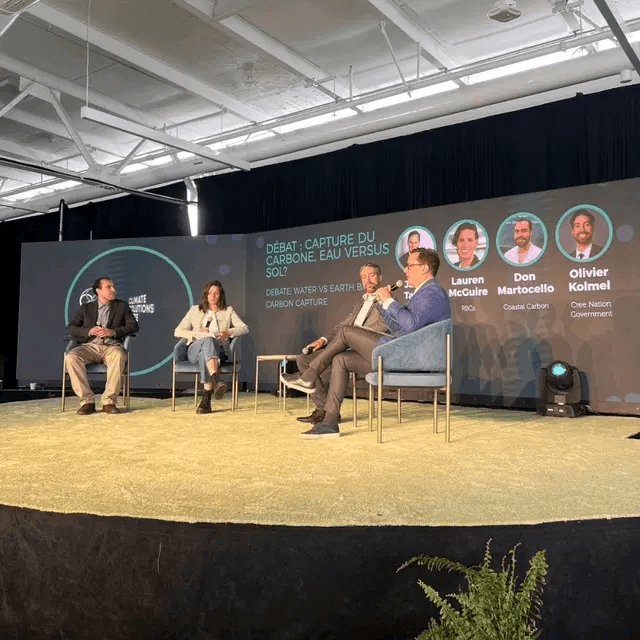
2024 Quebec Climate Solutions Festival
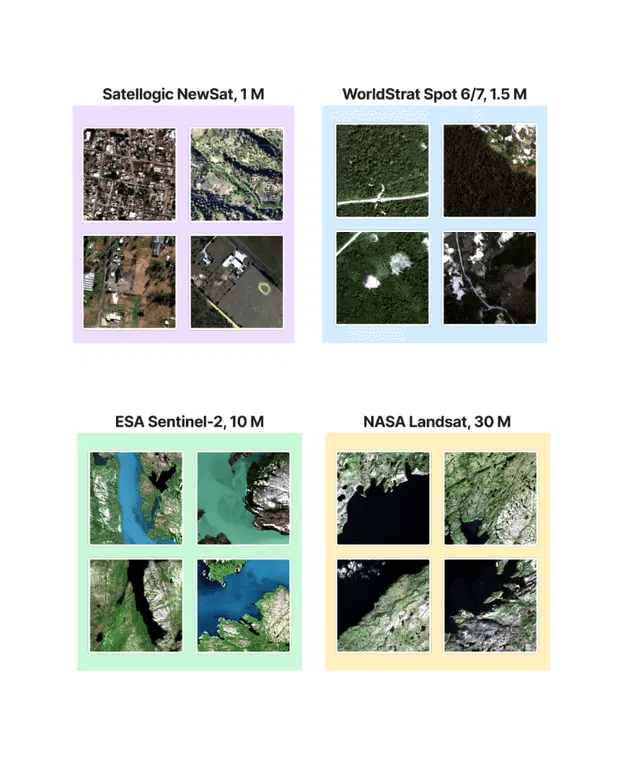
Unlocking the Power of Satellite Imagery with SeeFar: A Comprehensive Multi-Resolution Dataset
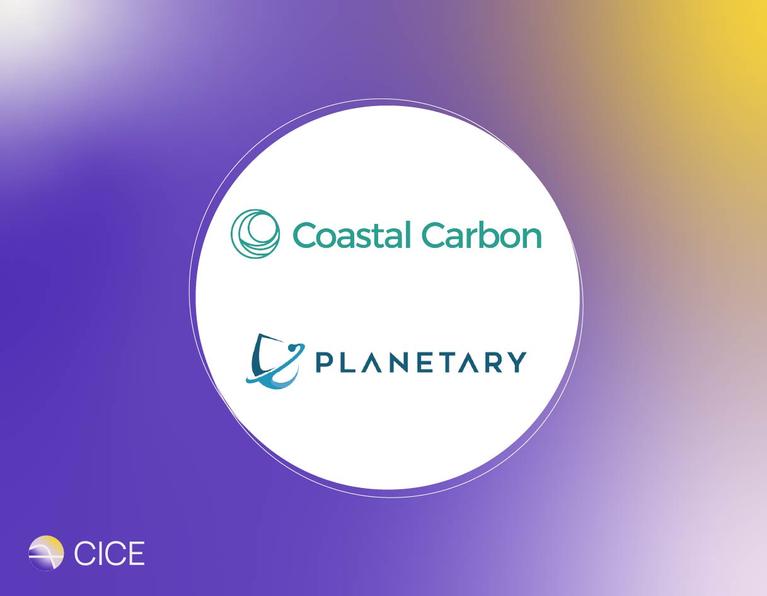
CICE Invests in the Future of Ocean Carbon Dioxide Removal
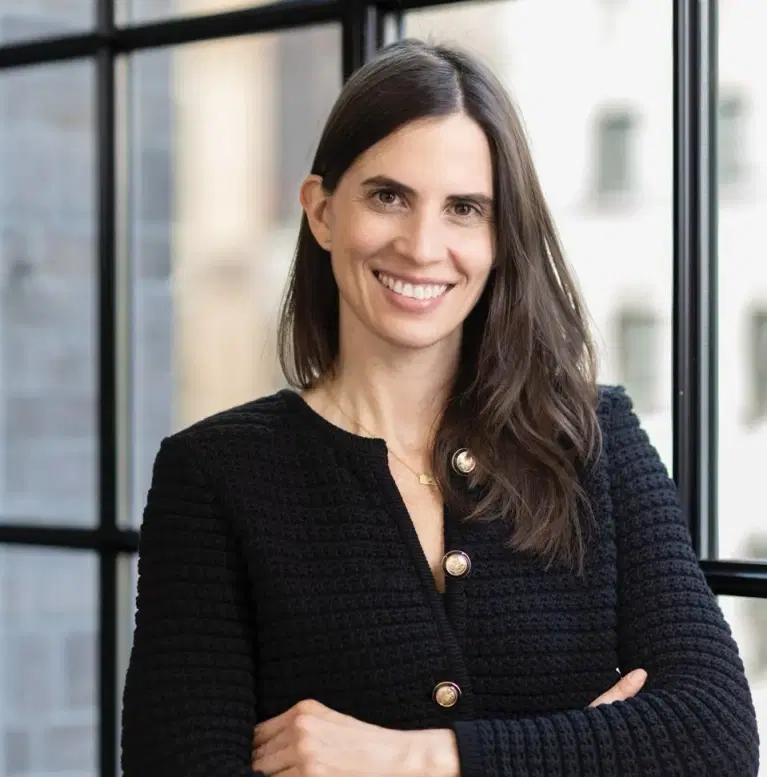
AWS Program Funds Proof-Of-Concept Efforts Of 4 Climate Startups
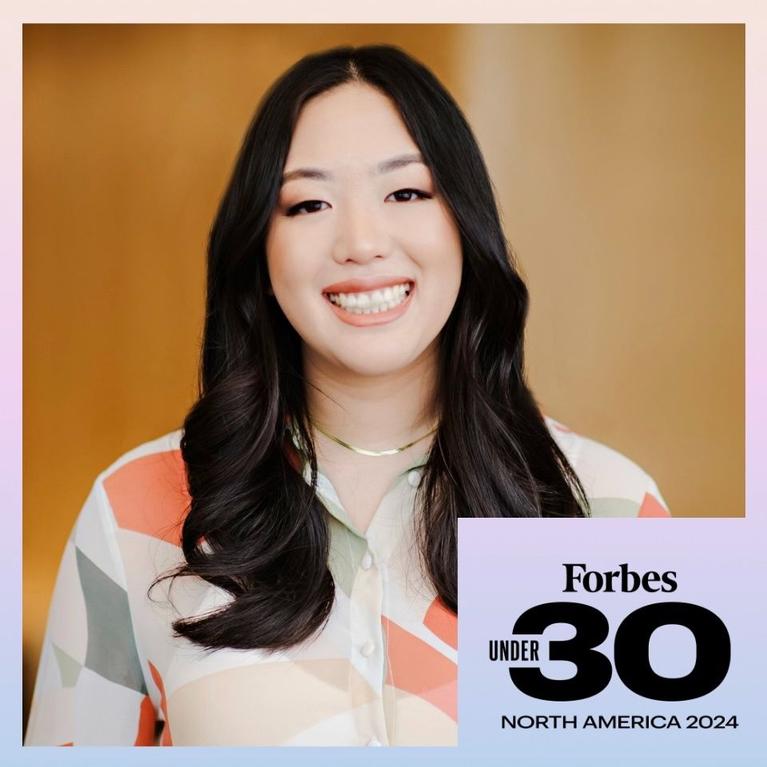
Coastal Carbon makes Forbes 30 under 30 Science 2024
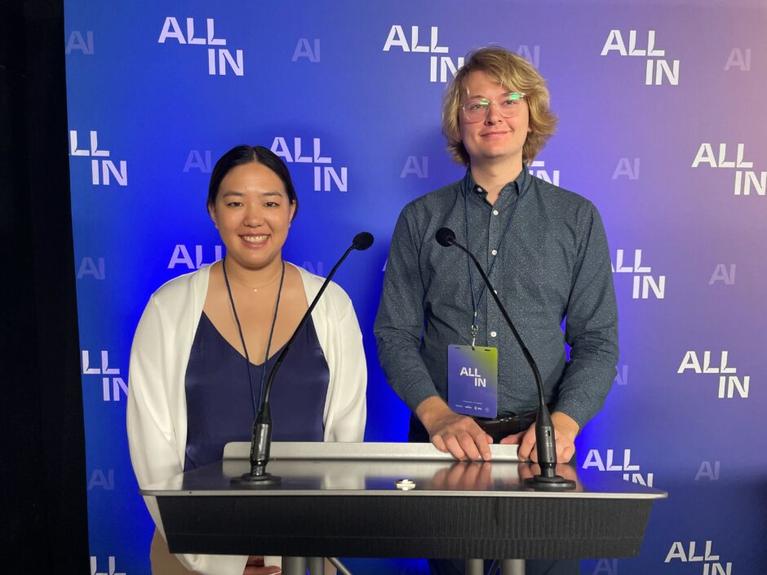
A fight against climate change is happening under water
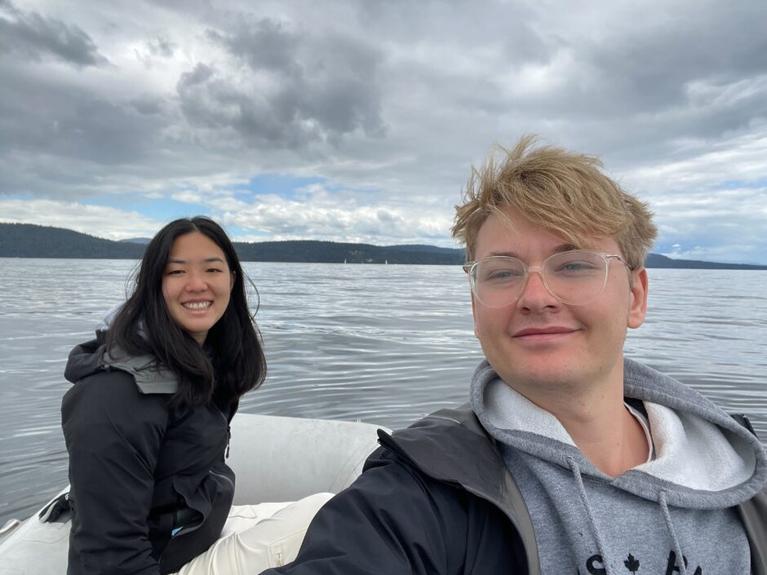
Exploring the underwater world to combat climate change
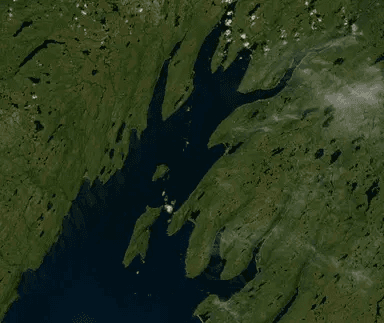
Ocean-Based Natural Climate Solutions: Seaweed